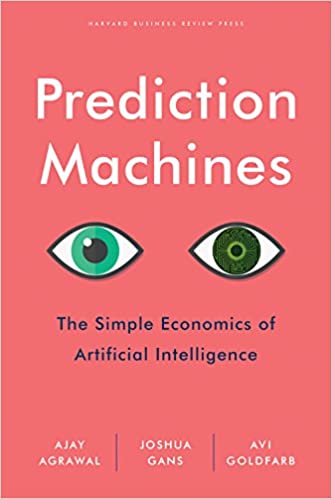
The Economics of AI
Ajay Agrawal, Joshua Gans, Avi Goldfarb and Catherine Tucker have organized a series of important and influential conferences on the economics of AI. The proceedings of the 2019 conference are open access and full of interesting persepctives.
Three of the conference organizers (Ajay Agrawal, Joshua Gans, and Avi Goldfarb) are all from the University of Toronto Rotman School of Management and published a 2018 book for general readers called Prediction Machines [1]. In this book, they view AI systems as prediction machines that dramatically lower the cost of predictions. In principle, as the cost of prediction falls, organizations can make more and better predictions, and hopefully better decisions. They are fundamentally focussed on the lower cost of predictions and how that is changing business.
There is absolutely no question that the price of predictions has been falling dramatically. I tend to look at this through a longer and broader perspective of commoditization. 1) The commoditization of compute has been driven by Moore’s Law over the past several decades; the commoditization of software has been driven by open source software, and, more recently, by cloud computing and Software as a Service (SaaS); and, 3) the commoditization of data has been driven by the exponential growth of new sources of data from the internet, from smart phones, and from IOT/OT devices. For over forty years, analytics has been at the intersection of these three trends and this confluence has been changing business over the same period. We just keeping calling it something different: data intensive statistics in the 1980s, data mining in the 1990s, predictive analytics in the 2000s, and AI in this decade. See [2].
From an economics perspective, the cost of predictions drops and continues to drop.
The Economic Challenges of AI
On the other hand, if you are launching an AI start-up or starting an AI initiative, it is important to look at some of the barriers in building a successful AI business. Martin Casado, a partner from Andreesen Horowtiz, and his colleagues have written a series of articles that are well worth reading on this topic, and the broader topic of the economics of AI, including:
- Martin Casado and Matt Bornstein, The New Business of AI (and How It’s Different From Traditional Software), February 16, 2020.
- Martin Casado and Matt Bornstein, Taming the Tail: Adventures in Improving AI Economics, August 12, 2020.
- Martin Casado and Peter Lauten, The Empty Promise of Data Moats, May 9, 2019
In these articles, there are insightful comparisons of AI start-ups compared to software as a service (SaaS) start-ups. Perhaps the most useful take home message for those not working in the industry is the following formula from [3]

The importance of this formula from Martin Casado and Matt Bornstein’s article “The New Business of AI” cannot be over emphasized. Although many AI start-ups, data science start-ups, and analytic start-ups may initially view themselves as a software start-up, they generally also end up involved with curating data and building models over the data; that is, they find themselves in a services business also.
The Four Elements of a Successful AI Business
Whether in the era of statistical modeling (80’s), data mining (90’s), predictive modeling (2000’s), or AI (2010’s), there have always been four critical elements.
Element 1. You need the data and the IT infrastructure to manage it.
Although data is being commoditized, getting the data you need to solve a problem that can be monetized is not always easy. Specialized IT infrastructure, may, or may not, be needed, depending upon the volume and velocity of the data.
Element 2. You need the expertise to clean the data and build the models.
This is often labor intensive and often involves exploratory data analysis, careful cleaning of the data, and experimentation to improve the model.
In addition, some models may take substantial amounts of data and substantial amounts of computing power, raising the cost of the model and its maintenance.
Element 3. You need software to build models.
Depending upon your solution, you may, or you may not, need to develop your own software.
We can summarize these three critical elements with a slight addition to Equation 1 to get Equation 2.

In practice, both Equations 1 and 2 miss a critical element that is at the core of most successful analytic companies.
Element 4. You need a business model that generates enough business value to justify the costs required to collect the data and build the model.
The point to keep in mind here is that services required to curate data and build models is often labor intensive and therefore the analytic model must generate enough business value to justify the costs to collect the data, curate the data, understand the data, build the model, improve the model, and manage the edge cases. This is not easy.
This brings us to Equation 3:

Although the economics of AI is dramatically lowering the cost of predictions, finding a business model to provide the foundation for a competitive and sustainable AI business still requires some effort. In addition, as pointed out in the articles by Martin Casado, the resulting AI business generally does not have the margins and scalability of a software company. These two basic facts have been the case for the past forty years.
In my book Developing an Analytic Strategy: a Primer [4], I take a slightly simpler perspective. These days with cloud computing and Software as a Service, the software is usually not the critical path. If you have the data, if you have the expertise, and if you have the business model, you can generally succeed. I call this the DEB Framework.
- Data. Is the data (“D”) required for your analytic strategy available? If not, do you have a realistic plan for getting it?
- Expertise. Is the expertise (“E”) required for processing and transforming your data available? Does this expertise include people who have developed, deployed, operated, and maintained similar models?
- Business model. Have you identified a business model (“B”) for monetizing the data or extracting the required value that is sustainable, provides compelling competitive advantages, and can be protected from current competitors and future new entrants into the market?
References
[1] Agrawal, Ajay, Joshua Gans, and Avi Goldfarb. Prediction machines: the simple economics of artificial intelligence. Harvard Business Press, 2018.
[2] Robert L. Grossman, The Structure of Digital Computing, Open Data Press, 2012.
[3] Martin Casado and Matt Bornstein, The New Business of AI (and How It’s Different From Traditional Software), February 16, 2020.
[4] Robert L. Grossman, Developing an Analytic Strategy: A Primer, 2020.
Notes About Links
There are no affiliate links in this post. and I get no revenue from the Amazon links. I do get a royalty from the sale of my books.