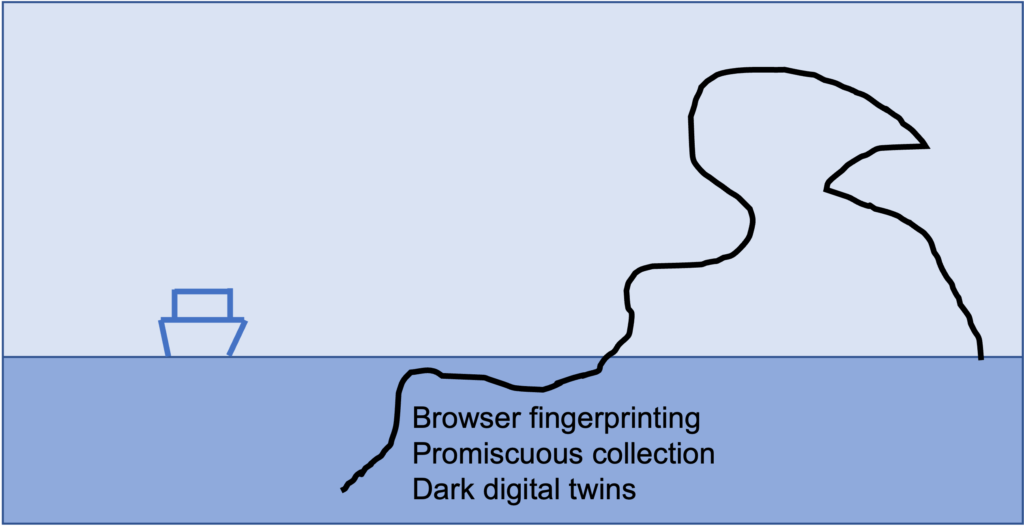
The term “dark patterns” refer to user interfaces that are deliberately designed to mislead users.
A good definition of the term dark patterns is contained in the article “Shining a light on dark patterns [1]:”
“Dark patterns are user interfaces whose designers knowingly confuse users, make it difficult for users to express their actual preferences, or manipulate users into taking certain actions [1].”
Getting the data you need for modeling is often the hardest part, and some organizations are tempted by using dark patterns to collect. In Chapter 6, of my Primer on developing an AI strategy, I cover several ways that data can be legitimately collected. In this post, I’m going to look at three dark patterns of data collection, in which the user is deliberately mislead. There are quite a few dark patterns of data collection, but here we will focus on just three of them.
Dark Pattern 1: Promiscuous Data Collection
This is one of the most common dark patterns used for collecting data. A good example of this approach is a weather application on your phone that provides the local weather but also collects your location data each day, extracts features from it to characterize your behavior, and then sells your location and behavior data to third parties. There is no standard name for this dark pattern, but I call it promiscuous data collection. Another good example is a game on your phone that collects and sells your location data. The terms of service that allow this are usually, but not always hidden in the click through agreement when you install the app. One of the definitions in the Oxford English Dictionary for promiscuous is: “Of an agent or agency: making no distinctions; undiscriminating,” and this certainly describe mobile applications that collect and sell data in this way.
Dark Pattern 2. Collecting Data Through Browser Fingerprinting
Since cookies can be deleting, advertisers and advertising networks have designed other ways to track your behavior. There are about 300,000,000 million people in the US, which is about 2^28, so about 28 bits of information are needed to identify someone in the US. Browser fingerprinting, also known as online fingerprinting or device fingerprinting, is the technique in which standard online scripts is used to collect information about the system you are using to browse the web, such as the extensions in your browser, the screen resolution and color depth of the system you are using, your time zone, the language you are using, etc. With answers to enough questions like these, a “fingerprint” can be formed that uniquely identifies a device used by an individual. For example, the number of bits of information in a user agent string that a browser provides varies, but 10 bits is a good average [2].
A good place to learn about browser and device fingerprinting is the the privacy education website Am I Unique. When I visited Am I Unique, my operating system, browser (Firefox, Chrome, Safari, etc.), browser version, time zone, and preferred language (all available from the browser when I visited the website) uniquely identified me via a browser fingerprint from 4.2M fingerprints in their database. Am I Unique collects 23 characteristics of your browser and device, although only five of these were needed to uniquely identify me.
I view browser fingerprinting as a dark pattern, because unlike with cookies, there is less you can do to block browser fingerprinting, although this has started to change. You can find a list of tools on the Am I Unique website that can provide some protection from browser fingerprinting.
Dark Pattern 3. Dark Digital Twins
Digital twins are “digital replications of living as well as nonliving entities that enable data to be seamlessly transmitted between the physical and virtual worlds [3]”. AI and deep learning are enabling the development of more functional and more powerful digital twins.
By a dark digital twin, I mean an AI application that either asks a series of questions or observes your behavior in one context with your consent, and then through any of several techniques, builds a profile of you and uses this profile in another context without your consent. As a simple hypothetical example, assume you are interacting with an AI application that is giving you wine recommendations, but the information used is then used in a dark digital twin to target you with vacation packages. Although this is pretty innocent example, as digital twin profiling technology improves, the use of dark digital twins is likely to become more and more disconcerting.
These days, it is more common for a machine learning or AI model built with user consent for one purpose is reused for another purpose without adequate user consent, but it is only a matter of time before the models became sophisticated enough to start acting as digital twins.
References
[1] Jamie Luguri and Lior Jacob Strahilevitz. “Shining a light on dark patterns.” Journal of Legal Analysis 13, no. 1 (2021) pages 43-109.
[2] Peter Eckersley, A Primer on Information Theory and Privacy, 2010, Electronic Freedom Foundation, retrieved from https://www.eff.org/deeplinks/2010/01/primer-information-theory-and-privacy on July 7, 2021.
[3] Abdulmotaleb El Saddik, Digital twins: The convergence of multimedia technologies, IEEE multimedia 25, no. 2 (2018) pages 87-92.