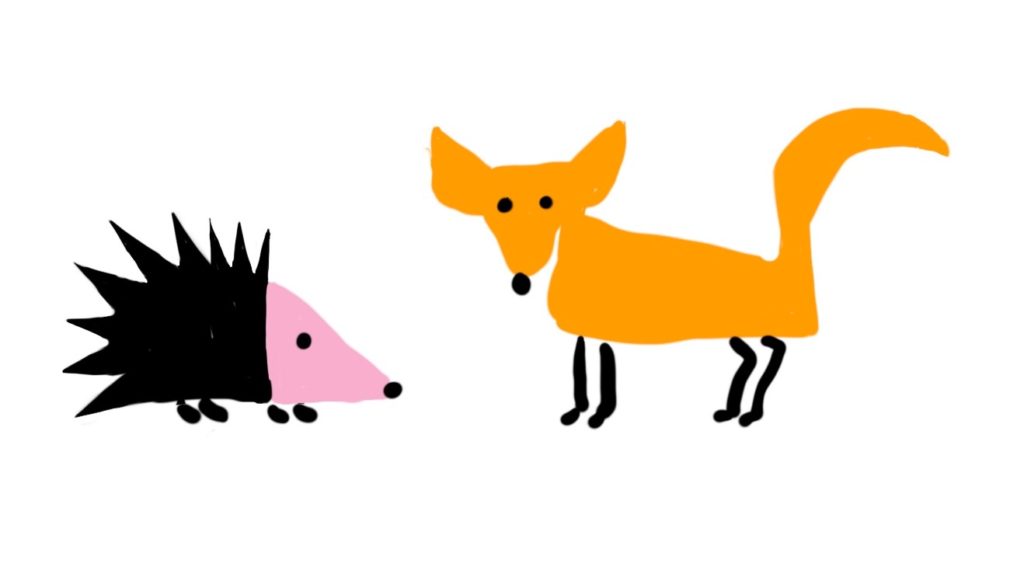
“The fox knows many things, but the hedgehog knows one big thing.” Archilochus, Greek poet, c. 680 – c. 645 BC
Question: “By the way, why did you make this classification [between hedgehogs and foxes]?” Isaiah Berlin: I never meant it very seriously. I meant it as a kind of enjoyable intellectual game, but it was taken seriously. Every classification throws light on something, this one was very simple.
For my January 2022 post, I want to return to return to the theme of last year’s January post about why a board should include someone with a good knowledge of analytic strategy. In this post, we will discuss different types of advisors about analysis and analytic strategy. It’s good to keep in mind the response of Isaiah Berlin points out when asked about his distinction between two types of thinkers (hedgehogs and foxes), “every classification throws light on something, [but] this one [is] very simple [1].”
In the January 2021, post I listed three reasons that a company should consider putting an expert on analytics on its board or one of its advisory boards:
- Analytic and AI strategy is too important for a company not to have someone with board level experience in this area.
- Board members with experience developing, deploying and operating complex analytic projects have critical experience using technology to innovate, not just operate.
- An analytic perspective for a board member helps with evaluating cybersecurity and digital transformation, both critical topics for many boards.
A year later, these three reasons are as important as ever. In this post, I’ll review five different types of advisors in general, and how this applies to advisors familiar with analytic strategy. It is important to note that an individual is often a blend of two or more of these types.
Type 1. The Problem Solver
The first type of advisor is a problem solver, who can listen carefully, think through a problem, challenge or situation thoughtfully and carefully, and then crystallize the essential issues, trade-offs and choices, so others most familiar with the facts can make a better decision. This type of advisor has experience, judgement, and often gravitas, but any particular domain knowledge is usually less important than their overall ability to pinpoint the essential issues and frame the tradeoffs that must be thought through. For simplicity, I call this type of advisor a problem solver, but they usually simply crystallize and frame a problem so others can more easily work out a good solution. This type of advisor does not rely on knowledge of any particular technical domain, and for this reason, can be valuably contribute to technical discussions over a long period of time, even as the underlying technology changes.
Type 2. The Connector
The second type of advisor is the connector who seems to know almost everyone of importance in a particular industry, has a track record of being helpful with introductions, and is happy to connect you as required. There are different types of connectors. Some will do an introduction, which will guarantee at least one (courtesy) meeting. Others will work to introduce two individuals that can be mutually valuable to each other and will continue to connect from time to time even if there is no immediate benefit.
Another type of connector is someone who is a trusted member of a group that has a shared common experience and whose members have gone on to different successes but stayed in touch. Examples of these types of groups include: the PayPal Mafia and the Fairchildren [2] that are the descendants of the Traitorous Eight.
Type 3. The Technical Expert
The third type is the technical expert, who has a deep understanding of a technical field, understands the economics and business value that the technical field, and has broad experience of the common problems and pitfalls and how to avoid them. This type of expert can save an enormous amount of time and costs for a company. Some challenges include that this type of expert may not always be the best at arguing his or her point of view and others, with less knowledge and experience, may be more pervasive. Another problem is that as technology changes and business and competitive environments change, the a particular advisor’s expertise may become less and less relevant.
A great example of an influential advisor who was Type 3 is John Tukey, one of the original data scientists [3], who came up with the idea of the fast Fourier transform (FFT) in a meeting of President Kennedy’s Scientific Advisory Committee in 1963 [4]. The discussion topic was the ability to verify a proposed United States/Soviet Union nuclear test ban. One idea was to analyze seismological signals obtained from off-shore seismometers that could be positioned to verify compliance with a test ban treaty. The seismometers would produce a lot of data and the FFT was one way to speed up the processing of the data. For an interesting history of the origins of the FFT and its acceptance by the scientific community, see [3]. For a broader overview of the FFT and its relevance to seismology, see [5].
Type 4. The Management Expert
The fourth type of advisor is the management expert, who can bring management expertise to a problem or opportunity. This is especially valuable when an outside perspective is needed. Sometimes these advisors may be put on a board because they are particularly familiar with certain management issues, such as corporate strategy, marketing, acquisitions, information strategy, change management, government sales, etc.
An archetype of a Type 4 advisor is Peter Drucker, who coined the term “knowledge worker” in the 1950’s and argued that knowledge workers were an important source of competitive advantage long before computers, information and digital transformation became commonplace [6, 7]. As another example, he argued that “purposeful abandonment” was a critical part of any business strategy. This is the argument that management focus requires a very purposeful pruning of business units and business activities that are no longer worthwhile spending resources to pursue, thus freeing up resources for more strategic activities. Although these observations sound quite familiar now, they were much less familiar when he first argued for them.
Type 5. The Trusted, Independent & Discreet Advisor
The fifth type of advisor is not required, and is not always present, but is the trusted and discreet advisor who is independent and often has a long relationship with those in the company or organization being advised.
An interesting example of the trusted and discreet advisor was Harry Hopkins, who was President Franklin Delano Roosevelt’s advisor and was influential in shaping the relationship between the United States and the United Kingdom in World War II.
Again, it is important to point out that an advisor is often a blend of two or more of these types.
Relevancy
Advisors seem to age at different rates. As long as they remain sharp and their expertise is still relevant to the company, the capabilities provided by the problem solver and the management expert remain relevant and useful. On the other hand, the value of the network of some connectors may become less relevant over time. Other connectors seem to have the almost magical ability to be personally acquainted with exactly the right individuals at the right time.
Finally, there are important differences in technical experts. Some have a deep and profound knowledge of certain technologies, such as data or analytics, and are comfortable using the current technical parlance and buzzwords For example, a technical expert of a certain age will move from framing technical discussions in the language of data mining (1990’s), to predictive analytics (2000’s), to data science (2010’s), to AI (2020’s). Others seem to stick with a particular framing of the problem, and although their advice is probably still relevant, it can seem dated and not relevant.
References
[1] Jahanbegloo, Ramin. 2007. Conversations with Isaiah Berlin. Halban. Kindle Edition. Location 2742.
[2] Laws, David. 2016. Fairchild, Fairchildren, and the Family Tree of Silicon Valley, December 20, 2016, retrieved from computerhistory.org on January 2, 2022.
[3] Donoho, David. 2017. 50 years of data science. Journal of Computational and Graphical Statistics, 26(4), pp.745-766.
[4] Cooley, James W. 1987. How the FFT gained acceptance. In Proceedings of the ACM conference on History of scientific and numeric computation, pp. 97-100.
[5] Rockmore, Daniel N. 2000. The FFT: an algorithm the whole family can use. Computing in Science & Engineering, 2(1), pp.60-64.
[6] Drucker, Peter F. 1959. Landmarks of Tomorrow. Harper, New York.
[7] Drucker, Peter F. 1992. The new society of organizations. Harvard Business Review 70(5) pp. 95-104.