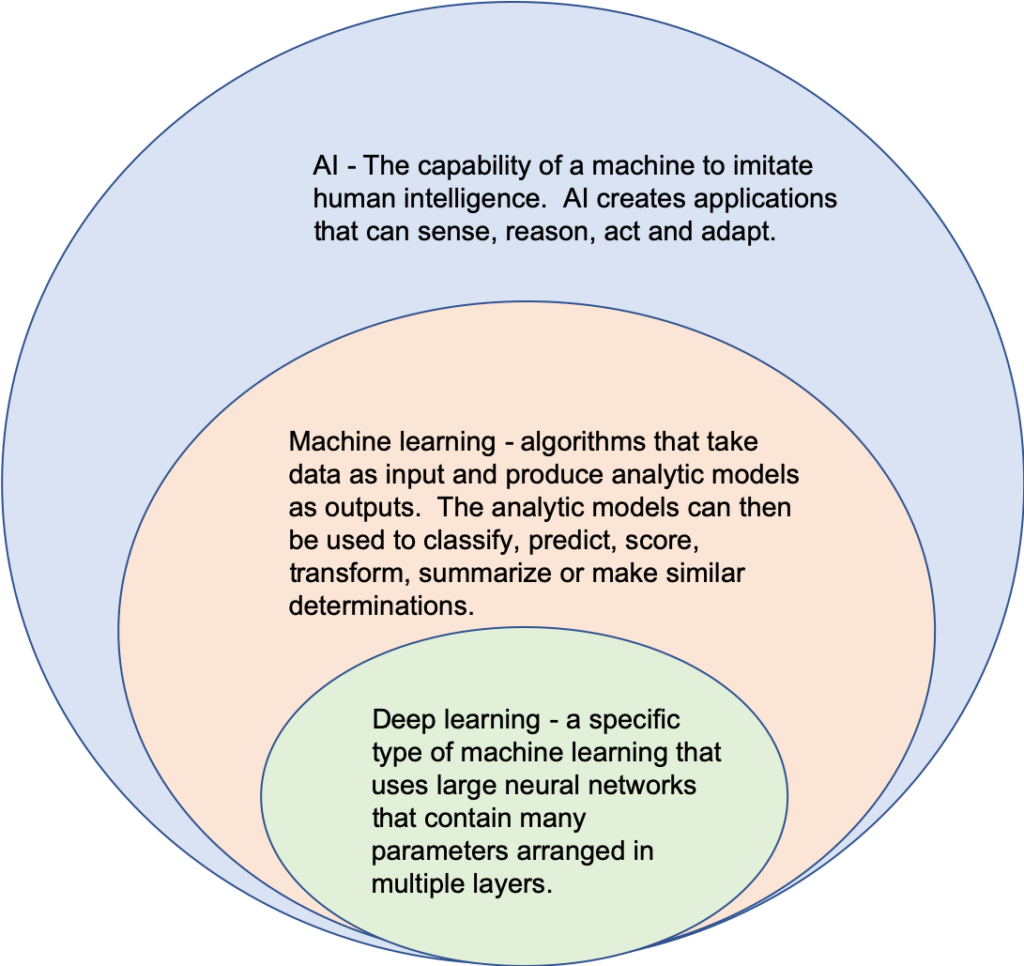
It is clear that artificial intelligence (AI) and machine learning (ML) are important, but with all the reports and with all the self-proclaimed pundits, it is easy to lose track of what is going on and what is essential. In this short overview, we go over 5 things that every senior executive should know about AI and ML.
The first two points discussed below may seem to be contradictory at first, but in fact are not. We will discuss both together, and, as we do, it may be helpful to slightly modify F. Scott Fitzgerald’s remark about holding two opposing ideas in mind as follows: “The test of a first-rate intelligence is the ability to hold two opposing ideas about an issue in your mind at the same time, and still retain the ability to make reasonable judgements about it.”
Here are the first two points:
Point 1. AI and ML is over hyped and a fair amount of what is described as AI and ML today is older technology that has been remarketed as AI.
Point 2. Over the past several years there have been some important advances in AI and ML and there is an argument to be made that “Data is the new oil and AI is the factory.”
Because of 2, it is important to take a new look at how you AI and ML can benefit your organization, if you haven’t done so recently. Because of 1), doing so can be challenging because of the hype and misinformation that is so rampant.
AI and ML have seen some important advances over the past few years. There are many reasons for this, but perhaps the most important are the following.
Three macro factors behind some of the recent advances in deep learning
- There is a lot more data available for machine learning and a lot more of it is labeled with the type of labels that many machine learning algorithms require.
- The underlying computing infrastructure (graphics processing units or GPUs) used by games turned out to be incredibly useful for machine learning, and even more specialized computing infrastructure for machine learning has been developed (for example, tensor processing units or TPUs)
- Over the past few years, there have been some nice algorithmic advances developed that leverage a) and b). These include a ML technique called transfer learning that take a ML model built for one problem and use in a component of a ML model for another problem.
On the other hand, it is just as important to keep in mind that AI is being seriously overhyped. It is relatively easy to raise venture funding in AI, which creates many companies that will not only not be around in a few years when their venture funding dries up, but aren’t producing much value in the near term, and are only greatly adding to the market clutter in the space. Last year in 2018, VCs invested a record $9.3B into US-based AI startups. This is over eight times the $1.1B invested in US-based AI startups five years ago in 2013 [1].
If you lead an organization, start with 2) and keep 1) in mind. If you lead a business unit that uses AI and ML as one of your enabling technologies, then you need to manage 1) and leverage 2).
It may be helpful to recall that we have seen this tension between real advances in building analytic models over large data and a hype driven by venture-backed startups twice before during the past 30 years. Although real advances were made in each period, there was also a hype cycle with most of the efforts not delivering much of lasting value.
- Hype cycle 1: Data mining and knowledge discovery (1995-2001)
- Hype cycle 2: Big data and data science (2010-2018)
- Current hype cycle: AI and machine learning (2016-present)
Point 3. With no new advances, new applications of AI and ML will be developed for some time and will continue to transform business.
There are an increasing number of applications in deep learning that are being developed, due primarily to the following factors.
Five factors that are driving new AI applications
- New sources of data, including location information and images from phones and data from the Internet of Things (IoT), operational technology (OT), online-to-offline (O2O), autonomous vehicles, etc.
- Easy access to powerful computing infrastructure due to cloud computing infrastructure containing GPU and TPU; as well as on-premise GPU clusters.
- The availability of large labeled datasets that are openly shared and readily available both for research and commercial applications.
- Powerful software frameworks that support machine learning in general and deep learning in particular.
- The unreasonable effectiveness of transfer learning and other algorithmic advances.
Unlike the prior periods of hype mentioned above, the current period has seen large investments in open source frameworks for machine learning and deep learning, including TensorFlow, PyTorch, and Keras. With the ability to leverage cloud computing containing GPUs and the availability of large labeled datasets, it is much easier than in the past periods to create ML and DL models given the right data. This is an important difference and one of the main reasons that the number of applications that are able to use ML and AI to provide meaningful performance improvements is significantly higher than in the 1995-2001 cycle and 2010-2018 cycle.
Because of this, we will probably see business and organizations continue to develop new deep learning applications for some time, even if there are no new algorithmic advances.
Point 4. Progress is very uneven.
The next point to keep in mind is that progress is quite uneven. It’s important to know which types of projects are likely to succeed and which ones are likely to fail. In this section, we describe three tiers of AI and ML projects. Tier 1 projects are likely to succeed if well executed. Tier 3 projects are likely to fail.
The most progress has been made in the Tier 1 – image, text and speech (ITS) processing. This is primarily for the five reasons a)-e) mentioned above, with the most important being the large amounts of labeled data that is available. Tier 2 applications requires simple judgements. This tier includes: spam detection, detecting fraudulent transactions, content recommendation and related problems. Tier 3 applications requires complex judgements. Examples of applications in this tier include: algorithmic hiring, recidivism prediction, and related applications. A recent study has shown that some algorithm hiring algorithms aren’t much better than random guessing [2].
Three Tiers of ML and DL Advances
- Image, text and speech (ITS) applications have seen significant improvements.
- Applications that require simple judgements have made good, but less dramatic improvements.
- Applications that require complex judgements and assessments have not made significant progress and significant progress shouldn’t be counted on in the near term.
Simple judgements are basically ML or other analytic models that produce scores with actions and rules within a well understood business process. As the judgements become more complex and action framework for actions becomes more complex, bias becomes more important, and distinguishing causality from association becomes more important. It’s important to note that ML has been used successfully in the second category for some time, including in cycles 1 and 2. The dramatic advances from the AI deep learning techniques has been largely focused in the first ITS category.
Arvind Narayanan has notes from his talk “How to Recognize AI Snake Oil [3]” that provides another perspective worth understanding in predicting which AI applications are likely to succeed and which are likely to failure. Narayanan distinguishes between three tiers of AI applications that overlap the categories above. The first category, which he calls perception is more or less the same as the ITS category above. Narayanan’s second category is automating judgements and his third category is predicting social outcomes. Recidivism prediction would be in his third category. His lecture notes [3] describe some of the successes in the first category and some of the snake oil fraud and failures in the third category.
Point 5. Deriving value from AI and ML projects is hard and many projects will fail to deliver any significant business value.
It’s helpful to keep in mind what I call the staircase of failure [4, Chapter 11]:
- Developing software is hard.
- Projects that require working with data are usually harder.
- Projects that require building and deploying analytic models are usually harder in turn.
If you think of this as a staircase, to deliver value, you must develop a software system, that processes data, uses the data to build models, and uses the models to produce scores that take actions that bring business value. In other words, you must climb the staircase to the top, which requires not only good technology, but also choosing the right problems, having good (usually labeled) datasets, and most importantly have a good analytic team [4, Chapter 12], a good project structure [4, Chapter 11], and a good way of using the outputs of the models to produce actions that bring business value [4, Chapter 13].
References
[1] CB Insights, The United States Of Artificial Intelligence Startups, November 26, 2019, retrieved from https://www.cbinsights.com/research/artificial-intelligence-startup-us-map/ on December 10, 2020. Also, see CB Insights, What’s Next in AI? Artificial Intelligence Trends, 2019.
[2] Manish Raghavan, Solon Barocas, Jon Kleinberg, and Karen Levy, Mitigating Bias in Algorithmic Employment Screening: Evaluating Claims and Practices, arXiv preprint arXiv:1906.09208, 2019.
[3] Arvind Narayanan, How to recognize AI snake oil, retrieved from https://www.cs.princeton.edu/~arvindn/talks/MIT-STS-AI-snakeoil.pdf on December 10, 2019
[4] Robert L. Grossman, The Strategy and Practice of Analytics, to appear.