“Success teaches us nothing; only failure teaches.”
Admiral Hyman G. Rickover, address to US Naval Postgraduate School, 16 March 1954
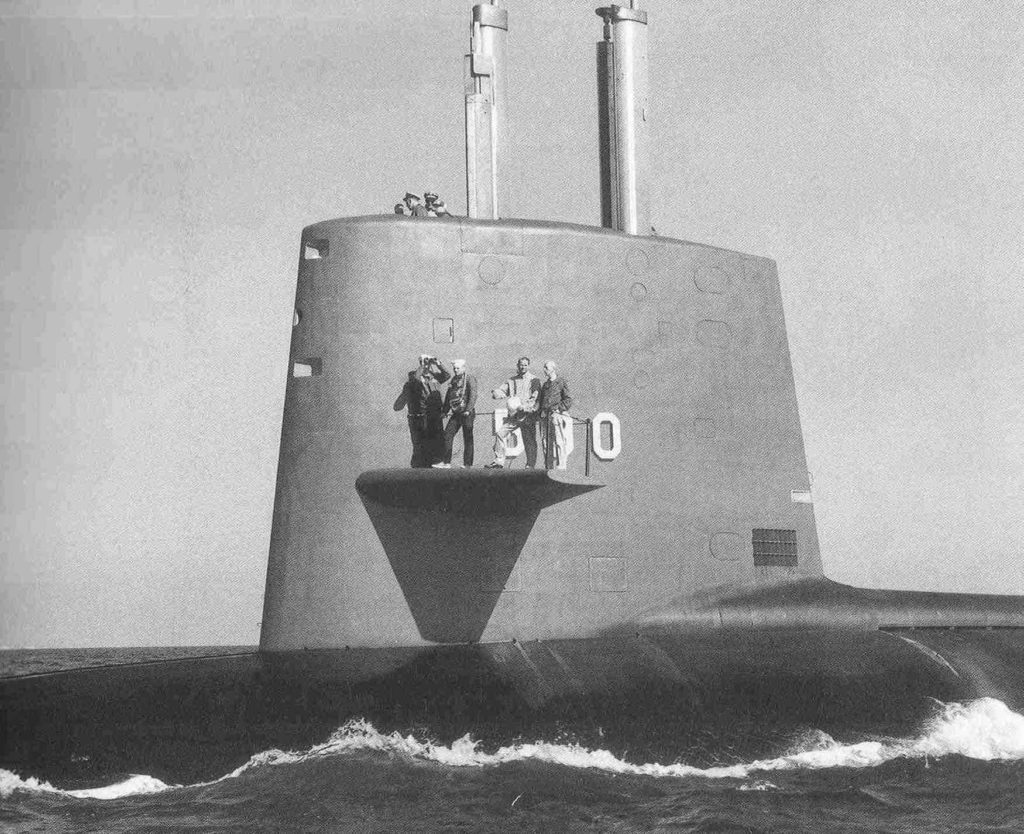
The Importance of Understanding Why Analytic Projects Fail
Although many writers have discussed the importance of understanding the reasons for project failures, I choose a quote from Admiral Hyman G. Rickover, the father of the nuclear submarine. In just a few years, from 1950-1953, not only did he develop a controlled nuclear reactor (nuclear explosions are not controlled and obviously not suitable for powering ships), but he miniaturized it so that it would fit on a submarine, and solved a host of technical problems so that the USS Nautilus submarine became the first submarine to cross the Atlantic without surfacing and without taking on any fuel [1]. Importantly, during Rickover’s command of the nuclear submarine program, there were zero reactor accidents [1].
One of Rickover’s rules was: “You must have a rising standard of quality over time, and well beyond what is required by any minimum standard [1].” These days we might phrase this as the need for process that continuously improves quality. In analytics and AI, we would apply this process of continuous improvement to ETL, to feature engineering, to model estimation, to refining the actions associated with the model outputs, and to quantifying the business value produced by the model.
The Staircase of Failure
One way of understanding why so many analytic and AI projects fail is what I call the staircase of failure. See Figure 1. For a machine learning or AI project to succeed, it must overcome many of the factors that cause many complex projects to fail, that cause many software projects to fail, and that cause many data warehousing projects to fail. I discuss this in Chapter 11 (Managing Analytic Projects) of my upcoming book The Strategy and Practice of Analytics.
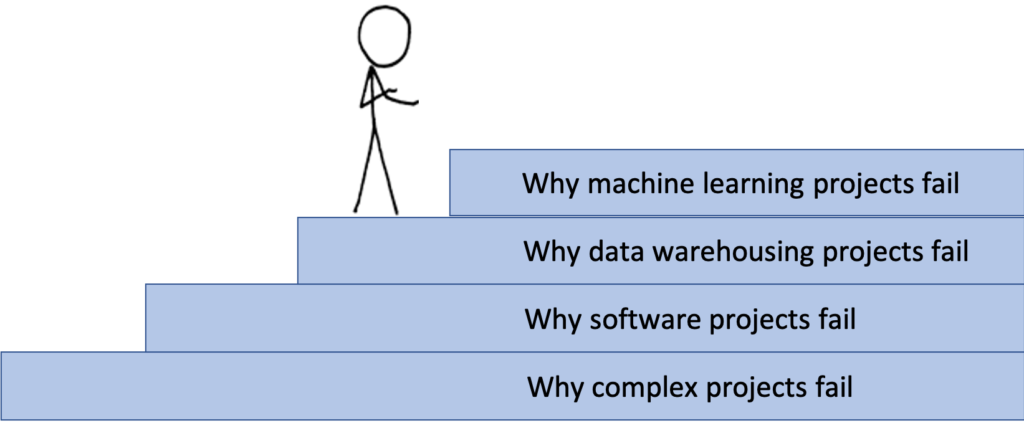
Five Dimensions of Risk
When managing an analytic project, there are five important dimensions of risk to manage [2]:
- Data risk
- Deployment risk
- Technical risk
- Team risk
- The risk that the model doesn’t produce the minimum viable value (MVV) required
I have talked about two of the biggest risks several times in this blog: data risk and deployment risk. Data risk is the risk that you won’t get the data that you need for the project and deployment risk is that you won’t be able deploy the model and take actions that produce the value needed to make the model successful. The SAM Framework is one way to manage the scores produced by the model, the associated actions that produce value, and measures to track the value produced.
Technical risk is the risk that the project doesn’t have the software or technical expertise required to acquire the data, manage the data, build the models, deploy the models, or support the actions required for the project.
The team risk is the risk that the team doesn’t have the required expertise or leadership to successfully complete the project.
Finally, MVV is the minimum viable value that the analytic model must generate for the project to be successful.
For complex projects, I plot these five dimensions in a radar plot and work to reduce the overall risk of the project along each of the dimensions over time [2]. See Figure 2.
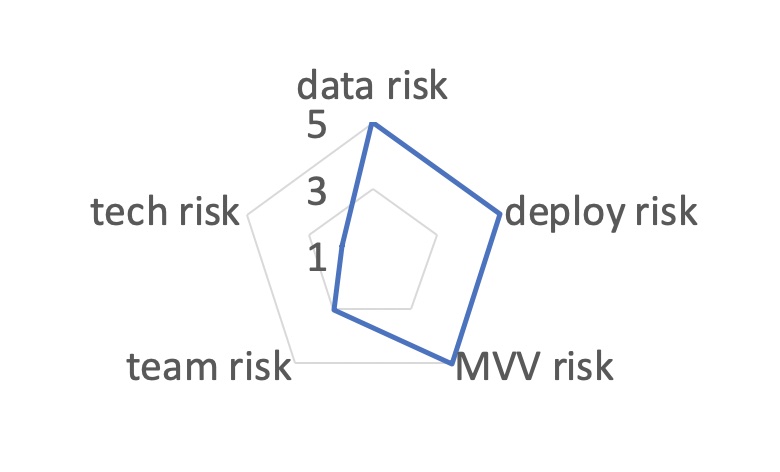
I’ll be speaking about managing analytic projects at the upcoming Predictive Analytics World.
Title: Why Do So Many Analytic and AI Projects Fail and What Are Some Frameworks for Improving the Odds of Success?
Event: Predictive Analytics World (PAW), Las Vegas, June 2, 2020 (now a virtual event)
Abstract: Many analytic models never get the data they need to be successful; many analytic models that do are never deployed successfully into operations; and, many deployed models never bring the value they promised to stakeholders. In this talk, I give a framework for those leading analytic or AI projects or interested in leading these types of projects in the future that improves the odds for overcoming these and related challenges.
References
[1] USN (Ret.) Rear Admiral Dave Oliver, Against the Tide: Rickover’s Leadership Principles and the Rise of the Nuclear Navy, Naval Institute Press, 2014.
[2] Robert L. Grossman, The Strategy and Practice of Analytics, Open Data Press, to appear.