According to a 2019 report from CB Insights [1], between 2010 and 2019 there were 635 AI acquisitions. The acquisitions break into three groups, as can be seen in the visualization below (Figure 1) from CB Insights. Facebook, Apple, Google, Microsoft, Amazon (FAGMA) and Intel accounted for 67 acquisitions, each making 7 or more acquisitions during the period 2010 to August 2019 (Group 1). Fifty two companies made between 2 and 6 acquisitions during this period (Group 2), and 431 companies made a single acquisition (Group 3).
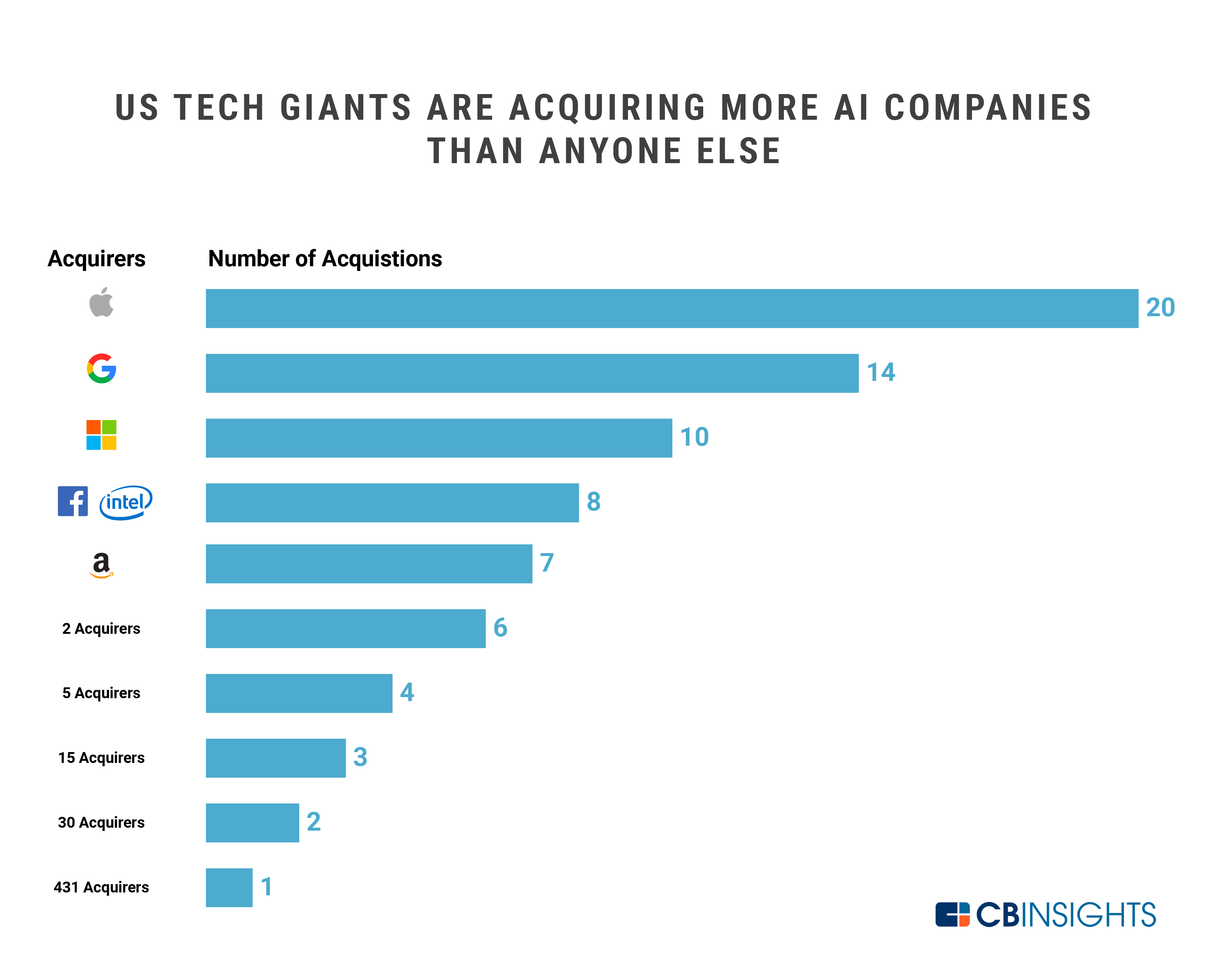
Reason 1. Analytic and AI strategy is too important for a company not to have someone with board level experience in this area.
Whether it is to ask critical questions about the single AI acquisition that 431 companies did between 2010 and 2019 or to ask critical questions about a company’s own analytic and AI efforts, a board member who has experience overseeing deployed analytic and AI applications is important.
It is important to note here the difference between someone who has experience in the entire life cycle of analytics from project start to deployment versus someone who only has experience developing analytic models. This is because most analytic projects don’t get deployed and don’t bring the expected value when deployed.
I taught a course at the University of Chicago’s Booth School of Business for three years called the Strategy and Practice of Analytics. One of my favorite case studies was HP’s acquisition of the AI company Autonomy in 2011 for $11.1 billion. As the New York Times reported a year later:
“Last week, H.P. stunned investors still reeling from more than a year of management upheavals, corporate blunders and disappointing earnings when it said it was writing down $8.8 billion of its acquisition of Autonomy, in effect admitting that the company was worth an astonishing 79 percent less than H.P. had paid for it [2].”
Reason 2. Board members with experience developing, deploying and operating complex analytic projects have critical experience using technology to innovate, not just operate.
All boards understand the importance of having board members that understand how to manage operations and risk to align with corporate strategy and drive financial performance. On the other hand, these days using technological innovation to align with corporate strategy and drive financial performance is also important. Successful senior leaders in analytics and AI generally have a deep understanding of technology innovation and how to use it to drive financial performance. See Figure 2.
In contrast, it is common for many CIOs to spend their time as CIOs managing IT operations, reducing IT costs, and using IT to quantify and control risks, rather than using IT to drive technology innovation and drive financial performance.
When successful, good analytic leaders find ways to use data, analytics and AI to change a company, not just run it. Having this perspective on a board is very valuable, as is experience with analytic projects that leverage continuous improvement and analytic innovation.
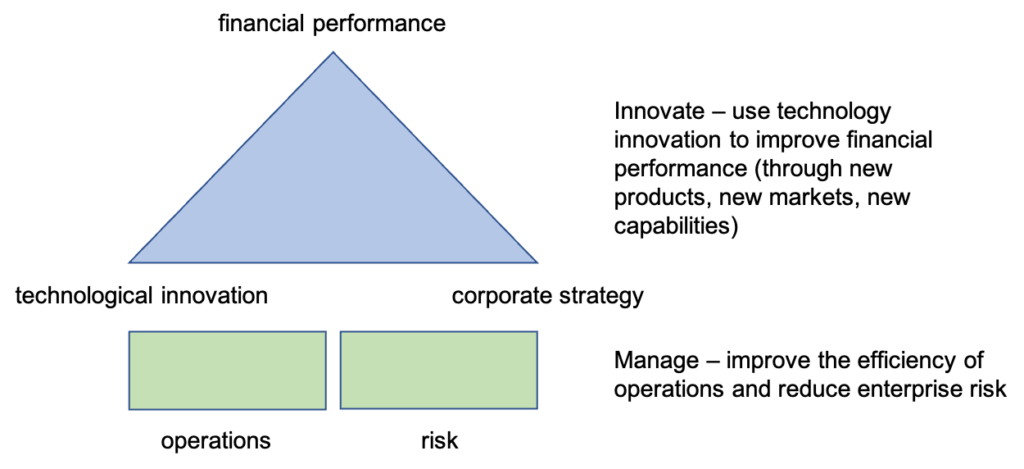
Reason 3. An analytic perspective for a board member helps with evaluating cybersecurity and digital transformation, both critical topics for many boards.
A 2017 Deloitte study found that:
“high-performing S&P 500 companies were more likely (31 percent) to have a tech-savvy board director than other companies (17 percent). The study also found that less than 10 percent of S&P 500 companies had a technology subcommittee and less than 5 percent had appointed a technologist to newly opened board seats. … Historically, board interactions with technology topics often focused on operational performance or cyber risk. The Deloitte study found that 48 percent of board technology conversations centered on cyber risk and privacy topics, while less than a third (32 percent) were concerned with technology-enabled digital transformation.” Source: Khalid Kark et al, Technology and the boardroom: A CIO’s guide to engaging the board (emphasis added) [3].
A senior analytics executive with experience supporting cybersecurity is a double win for a board. Even without this experience, behavioral analytics plays an important role in the cybersecurity for a large enterprise, and senior analytics executives almost always have experience in behavioral analytics.
The remote work caused by the COVID-19 pandemic has accelerated the importance of board level understanding of digital transformation. As a Wall Street Journal article from October 2020 states it:
“If you didn’t have a digital strategy, you do now or you don’t survive,” said Guillermo Diaz Jr. , chief executive officer at software firm Kloudspot, and a former chief information officer at Cisco Systems Inc. “You have to have a digital strategy and digital culture, and a board that thinks that way,” he said. Source: Angus Loten, Many Corporate Boards Still Face Shortage of Tech Expertise, Wall Street Journal [4].
It is hard to image a digital strategy without an analytic strategy. Chapter 8 of my Developing an Analytic Strategy: A Primer [5] describes seven common strategy tools that can be easily adapted to develop an analytic or AI strategy, including SWOT, the Ansoff Matrix, the experience curve and blue ocean strategies.
References
[1] CBInsights, The Race For AI: Here Are The Tech Giants Rushing To Snap Up Artificial Intelligence Startups, CB Insights, September 17, 2019. Retrieved from: https://www.cbinsights.com/research/top-acquirers-ai-startups-ma-timeline/.
[2] James B. Stewart, From H.P., a Blunder That Seems to Beat All, New York Times, Nov. 30, 2012. Retrieved from: https://www.nytimes.com/2012/12/01/business/hps-autonomy-blunder-might-be-one-for-the-record-books.html
[3] Khalid Kark, Minu Puranik, Tonie Leatherberry, and Debbie McCormack, CIO Insider: Technology and the boardroom: A CIO’s guide to engaging the board, Deloitte Insights, February 2019. Retrieved from: https://www2.deloitte.com/us/en/insights/focus/cio-insider-business-insights/boards-technology-fluency-cio-guide.html
[4] Angus Loten, Many Corporate Boards Still Face Shortage of Tech Expertise: But more CIOs are expected to earn a seat as the pandemic forces companies to lean on digital, Wall Street Journal, Oct. 12, 2020. Retrieved from: https://www.wsj.com/articles/many-corporate-boards-still-face-shortage-of-tech-expertise-11602537966