In this post, we continue our profiles of individuals who have made contributions to the practice of analytics by discussing the life and work of W. Edwards Deming. As I will explain below, it may be helpful to think of statistical quality control in the 1950’s and 1960’s as playing some of the same role that predictive analytics did in the last decade and that AI does in this decade.
Deming made a number of contributions to the practice of analytics, but in my opinion, three of the most important were:
- Developing and refining a process loop (Deming Cycle) for improving quality.
- Organizing Walter Shewhart’s notes about statistical process control and editing them to produce an influential book that was published in 1939. The book is still available as a Dover reprint for less than $15 and still worth reading.
- Bridging sampling methodology with the practical practice of statistical quality control and teaching thousands of people about it in seminars, tutorials, and consulting engagements, including in a series of influential seminars in Japan after World War II.
Why does Deming and his influence on statistical process control in the 40’s – 80’s matter today? The reason is simple. We have good software frameworks for building analytic models and many textbooks about deep learning, but little practical advice about how to build high quality systems with embedded deep learning. To say it simply, we could use some Demings for deep learning.
In this post, I’ll cover four facts about Deming that provide a perspective on his importance on the practice of analytics. From the viewpoint of the analytic diamond, Deming had a deep knowledge and important insights both about analytic modeling (the technical foundations and methodology) and analytic operations (part of the practice of analytics). But this is not the reason that most people in analytics know his name today. First, he was in the right place at the right time –with the US government during the World War II where quality was critical for the industrial productivity required to beat the Axes powers and in Japan during its postwar reconstruction where quality was critical for pivoting Japanese industry towards high quality manufacturing. Second, his emergence as a guru was catalyzed by a 1980 NBC documentary about him. Prior to the documentary, he was relatively unknown.
Understanding the contributions of W. Edwards Deming to the practice of analytics is not as simple as it as first appears. The British Library has a nice balanced assessment [1]:
"William Edwards Deming (1900-1993) is widely acknowledged as the leading management thinker in the field of quality. He was a statistician and business consultant whose methods helped hasten Japan’s recovery after the Second World War and beyond. He derived the first philosophy and method that allowed individuals and organisations to plan and continually improve themselves, their relationships, processes, products and services." Source: W. Edwards Deming, https://www.bl.uk/people/w-edwards-deming.
Deming’s Early Career and Two Defining Events
Deming (1900-1993) received a M.S. from the University of Colorado, Boulder in 1924 in mathematics and mathematical physics and a PhD from Yale University in 1928 in mathematical physics [7].
The first defining event in Deming’s education was the time he spent over the summers of 1925 and 1926 as an hourly worker at Western Electric’s Hawthorne Works in Cicero, near Chicago [7]. Telephone equipment was mass produced at Hawthorne Works, which employed over 45,000 workers in factory conditions that were extremely monotonous. Deming’s time at the Hawthorne Works gave him first hand knowledge about assembly lines and the complex interaction of mechanical processes, human processes, and the different types of variations that resulted.
Hawthorne Works was the home for several critical studies in industrial management. During 1924-1932, what became known as the Hawthorne Experiments on industrial productivity were conducted there. Although, Deming was not directly involved in them, one of the results of the Hawthorne Experiments and similar work was the emergence of the human relations movement within management studies that balanced Frederick Taylor’s scientific management movement. (Note that the Hawthorne Experiments were related to, but different, then what later became known as the Hawthorne Effect [9].)
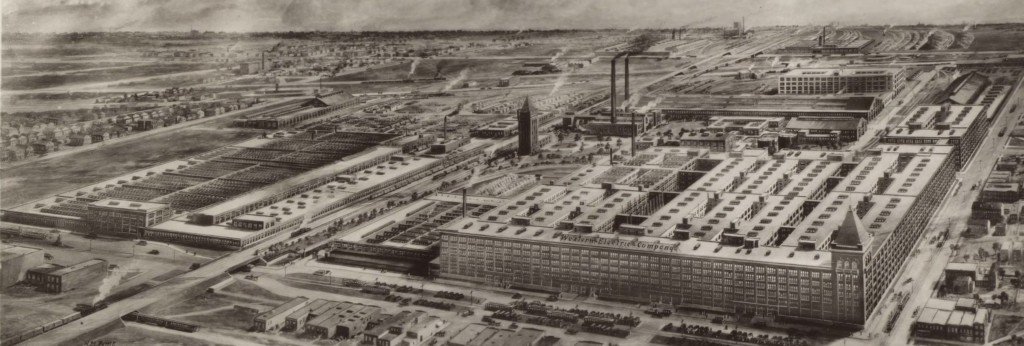
In 1927, Deming took a job at the US Department of Agriculture (USDA). While there, he was introduced to Walter A. Shewhart of the Bell Telephone Laboratories. The second defining event of his early career is that Deming edited a series of lectures delivered by Shewhart at USDA. This became the book by Shewhart called Statistical Method from the Viewpoint of Quality Control that was published in 1939 [6]. The material covered in the lectures included the core concepts of statistical quality control and the control chart.
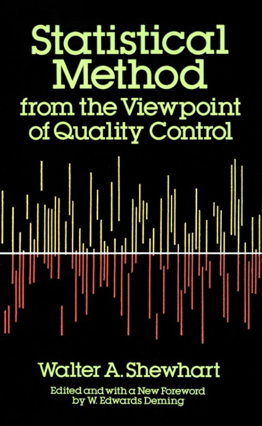
Deming’s Technical Mastery of the Statistical Process Control
Fact 1. Deming had a deep technical understanding of the field of statistical process control. Deming understood all the mathematics and statistical required and spent over two decades practicing statistical process control. He collected and organized this technical material into a 602 page book called Some Theory of Sampling that was published by John Wiley & Sons in 1950 [2]. The book was reviewed in 1951 in the Journal of the American Statistical Association:
The information in this book is so extensive that the presentation may appear bewildering at first glance. But once the reader gets acquainted with its contents, it becomes clear that the topics are developed logically and systematically. It seems likely that for some time to come this book will be the "bible" of sampling statisticians [4].
The information in this book is so extensive that the presentation may appear bewildering at first glance. But once the reader gets acquainted with its contents, it becomes clear that the topics are developed logically and systematically. It seems likely that for some time to come this book will be the “bible” of sampling statisticians.
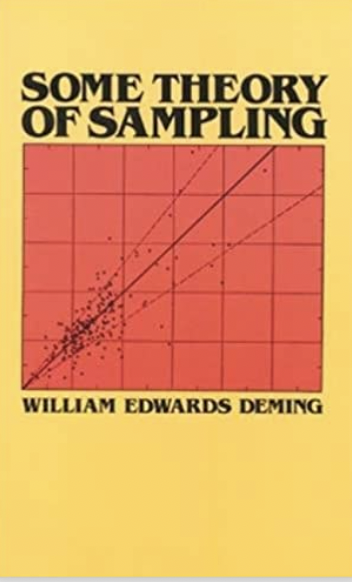
Deming as a Practitioner
Fact 2. Deming was a great practitioner of statistical quality control. Deming gained over a decade of practical experience at the Department of Agriculture, Census Bureau and Department of Defense with statistical process control before he advised the Japanese manufacturing community during the reconstruction after World War II.
Deming as a Teacher – The Red Beads Experiment
Fact 3. Deming was a great teacher.
The Red Beads Experiment was a three day experiment that Deming used as the core in many of his tutorial introductions to statistical process control. The description here follows [5]. Deming began using the red bead experiment in the early 1980’s.
The Red Bead Experiment uses a control chart (also known as a process behavior chart) to show that even though a 'willing worker' wants to do a good job, their success is directly tied to and limited by the nature of the system they are working within. Real and sustainable improvement on the part of the willing worker is achieved only when management is able to improve the system, starting small and then expanding the scope of the improvement efforts. Source: Red Bead Experiment, accessed from: https://deming.org/explore/red-bead-experiment/
The red bead experiment uses the following:
- box of wooden beads, with 3,200 white beads and 800 red beads
- a paddle with with fifty bead size holds
- a box for mixing the beads
- six workers
- two inspectors who count the beads produced using the paddles
- a chief inspector who verifies the counts
- an accountant who records the counts
- and a customer who accepts only white beads
The goal is for each worker to produce fifty white beads per day. The process starts by mixing the beads using the two boxes. Next each of the six workers dips the paddle into the larger box without shaking it, carries the paddle to each inspector for counting and verification, and then dumps the paddle back into the larger box, so the next worker can repeat the process.
After each of the six workers completes this process (thought of as corresponding to one day), the manager makes decisions about how best to reward the successful workers (those that produced the most white beads) and how to put in place performance improvement plans for the least successful workers (those whose work had the most red beads). Of course, who produces the most white beads and the most red beads is a random process, and nothing can change until the production line / process itself changes.
By carrying out the process for four rounds (corresponding to days) and analyzing the experiment over days of instruction provided an opportunity for thoroughly covering everything from the sampling approach and how to manage, to the variation between workers, and how what appeared to be differences in the talent of the scoopers was nothing more than normal variation.
Deming used 4 paddles over the 45 years that he used the red bed experiment in his teaching and kept track of the mean number of red beads for each paddle. It was 11.3, 9.6, 9.2 and 9.4 [3].
Those that took Deming’s three day course learned a lot from them. These days, when instruction is so different, it might be hard to understand this, but Deming intermixed sampling theory from binomial distributions, with understanding natural variation, with understanding how to manage and reward workers, with advice how to diagnose and improve industrial systems and processes. In our language, it intermixed both analytics models/analytic methodologies with the practice / management of analytics, something that is rare today, despite the number of data science boot camps.
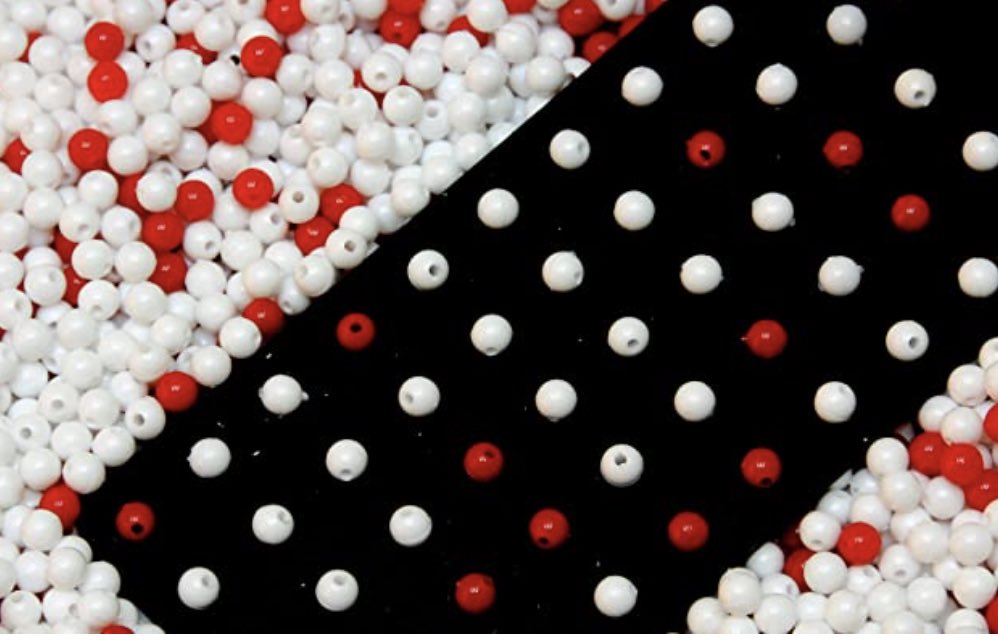
The Role of the NBC Documentary “If Japan Can, Why Can’t We?”
Fact 4. Deming was in the right place at the right time, and the 1980 NBC documentary was a catalyst. A critical role was played by the 1980 NBC documentary “If Japan Can, Why Can’t We?” A very thoughtful discussion of the importance of this documentary is provided in the analysis by William M. Tsutsui in [8].
Immediately after the documentary, CEOs from US companies wanted Deming to teach their workers how to improve quality, and his fame grew substantially [8].
References
[1] British Library, W. Edwards Deming, https://www.bl.uk/people/w-edwards-deming, accessed on May 10, 2021.
[2] Deming, W. Edwards, Some Theory of Sampling, John Wiley & Sons, 1950. Reprinted Dover Publications, 1984.
[3] Deming, W. Edwards. 1993. The New Economics for Industry For Industry, Government & Education. MA. Massachusetts Institute of Technology Center for Advanced Engineering Study. Chapter 7.
[4] Frankel, Lester R. Journal of the American Statistical Association 46, no. 253 (1951): 127-29.
[5] Martin, James R., What is the Red Bead Experiment, https://maaw.info/DemingsRedbeads.htm, accessed on May 12, 2021.
[6] Shewart, Walter, Statistical Method from the Viewpoint of Quality Control, US Department of Agriculture, 1939.
[7] The W. Edwards Deming Institute, Timeline, accessed from https://deming.org/timeline/ on May 2, 2021.
[8] Tsutsui, W.M., 1996. W. Edwards Deming and the origins of quality control in Japan. Journal of Japanese Studies, 22(2), pp.295-325.
[9] Wickström, G. and Bendix, T., 2000. The “Hawthorne effect”—what did the original Hawthorne studies actually show?. Scandinavian journal of work, environment & health, pages 363-367.