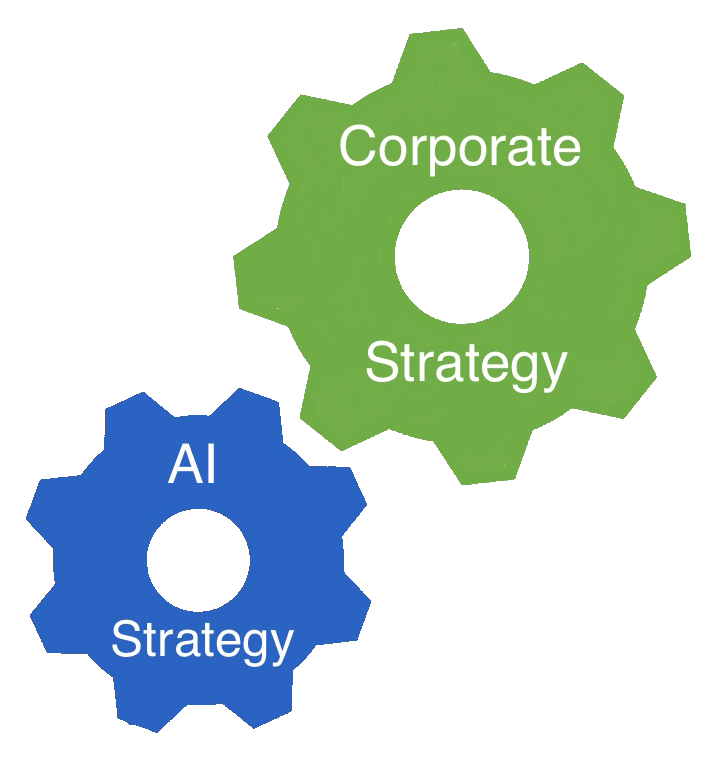
Although the name of the blog is Analytic Strategy, we haven’t discussed analytic strategy per se in a while, and it is time to return to the topic. An analytic strategy is a functional component of a corporate or organizational strategy. Other functional component strategies include: a product strategy, a marketing strategy and an IT strategy. In this post, we discuss four points of view for developing a corporate or business strategy in general that might be helpful as you develop an analytic or AI strategy component strategy.
Strategy through systematic planning
What is sometimes called the classical approach to business strategy is focused on profitability and using systematic planning to achieve it. An exemplar of this approach was the strategy pursued by General Motors under the leadership of Alfred Sloan as President of the company from 1923-1943 and as Chairman of the Board from 1937-1956. Under his leadership, GM sales grew over 10 percent per year for thirty-three years, and by 1956 GM was double the size of the next largest company [1]. Profits in 1955 were 50% higher than those at each of the next three largest companies [1]. The importance of focusing on profitability achieved by systematic planning was codified during the 1960’s with the writings of Alfred Chandler [2], Alfred Sloan [3] and Igor Ansoff [4].
Strategy through competitive positioning
One of the most influential contemporary thinkers about business strategy is Michael Porter, who is the Bishop William Lawrence University Professor at Harvard Business School. His 1980 book Competitive Strategy [5] book was selected as one of the most influential management books of the 20th century by the Academy of Management [6].
Porter views strategy as a competition between firms in a market and recommends positioning a firm within a sector using a framework called the five forces so that there are structural barriers allowing the firm to sustain above average profits over a long a time period [5]. The five forces are competitive rivalry, the bargaining power of suppliers, the bargaining power of buyers, the threat of substitution, and the threat of new competitors.
In 1983, Michael Porter co-founded a consulting company called the Monitor Group to help companies implement his view of competitive strategy [6]. It’s important to keep in mind that external forces can overwhelm even the best strategy. The Monitor group was quite successful for over 25 years, but struggled during the 2008 financial crisis, declared bankruptcy in 2012, and was acquired by Deloitte Consulting in 2013 [7].
Strategy through CEO leadership and management excellence
Around the same time, Tom Peters and Robert H. Waterman, Jr. wrote In Search of Excellence [8], which was published in 1982 and sold over 3 million copies in its first four years. In this book, they told stories about how companies achieved excellence and organized the stories around what they called their 7S Framework, which consisted of strategy, structure, systems, staff, style, skills, and shared values. The focus was less on the economics of competitive positioning and more on the people, processes, culture, and leadership, with the emphasis on the leadership, that could lead to excellence.
Most people views of complex subjects like strategy are shaped through stories, and good stories about CEOs are quite sticky [9].
Strategy through sustainable technological innovation
Beginning in the late 1990’s and early 2000’s, a different view of strategy began to emerge that used technology, innovation, and large amounts of digital data to steadily improve a product or service. A particularly powerful approach was to create digital platforms that linked producers and consumers [10]. This data driven and analytics-based approach led to the success of companies like Amazon (founded 1994), Google (founded 1998), and Facebook (founded 2004). The technology was enabled by software, and in the words that captured this insight, “software began eating the world [11].”
You can think of the recent advances in deep learning and AI as a natural trajectory that began in the 1990’s and has continued as Moore’s law led to more computational power; the growth of the internet, mobile devices and the internet of things (IoT) led to more data; and powerful open source software frameworks began transforming processes [12].
The Practice of Management by Peter Drucker
This post started with Alfred Sloan and GM and it’s a good way to end it. Peter Drucker was invited by General Motors to spend the two year period 1943-1945 studying the company, which led to his 1946 book The Concept of the Corporation [13]. This book was one of the early books to focus on the corporation as an entity worth studying and helped popularize viewing strategy through the lens of systematic planning, the first tradition described above. Alfred Sloan’s book My Years at General Motors can be viewed in part as a counterpoint to Drucker’s book [14]. For those interested in the history of strategy, both books are still worth reading today, as well as Drucker’s 1954 book The Practice of Management [15].
On the other hand, in the current era, digital technology has fundamentally changed how we work, manage, plan, live, and play, and has created new capabilities that in turn has created new businesses and new business models. For this reason, with the commoditization of data, computing power, network bandwidth, and software [12], it’s a good time to rethink and reinterpret your business strategy.
Developing an AI or analytic strategy
I wrote a short primer about Analytic Strategy that discusses how to develop an AI or analytic strategy from several different points of view. It is designed to be self-contained and provides short introductions to both analytics and strategy
The primer covers seven standard strategy tools and frameworks that can be adapted to analytics and AI, including: analytic SWOT, Analytic Ansoff Matrix, Porter’s Five Forces, Blue Ocean Analysis, the Analytic Experience Curve, and PESTEL analysis.
The book also covers specialized tools and frameworks that I have developed to support analytic strategy, including the Analytic Diamond and the Analytic Value Chain.
You can buy Developing an AI Strategy: A Primer online.
References
[1] Hoover, Gary, The Greatest Businessman in American History: Alfred P. Sloan, Jr., American Business History Center, November 4, 2021. Retrieved from https://americanbusinesshistory.org/the-greatest-businessman-in-american-history-alfred-p-sloan-jr.
[2] Chandler, Alfred D. Strategy and structure, MIT Press, 1962
[3] Sloan, Alfred My Years with General Motors, Doubleday & Company, 1963
[4] Ansoff, Igor H, Corporate Strategy, 1965
[5] Porter, Michael, Competitive Strategy, Free Press, New York, 1980.
[6] Bedeian, Arthur G., and Daniel A. Wren. “Most influential management books of the 20th century.” Organizational Dynamics 3, no. 29 (2001): 221-225.
[7] Denning, Steve, What Killed Michael Porter’s Monitor Group? The One Force That Really Matters, Forbes, November 20, 2012.
[8] Peters, Thomas J. and Robert H. Waterman, In Search of Excellence: Lessons from America’s Best-Run Companies, Harper & Row, 1982
[9] Heath, Chip and Dan Heath, Made to Stick: Why Some Ideas Survive and Others Die, Random House, 2007.
[10] Parker, Geoffrey G., Marshall W. Van Alstyne, and Sangeet Paul Choudary. Platform Revolution: How Networked Markets Are Transforming the Economy? and How to Make Them Work for You. WW Norton & Company, 2016.
[11] Marc Andreessen, Why Software Is Eating the World, Wall Street Journal, August 20, 2011.
[12] Grossman, Robert, The structure of digital computing: from mainframes to big data. Open Data Press, 2012.
[13] Drucker, Peter F., Concept of the Corporation, John Day, 1946
[14] Kay, John, The concept of the corporation, 2017, retrieved from https://www.johnkay.com/2017/03/16/the-concept-of-the-corporation/
[15] Drucker, Peter, The Practice of Management, Harper, 1954.