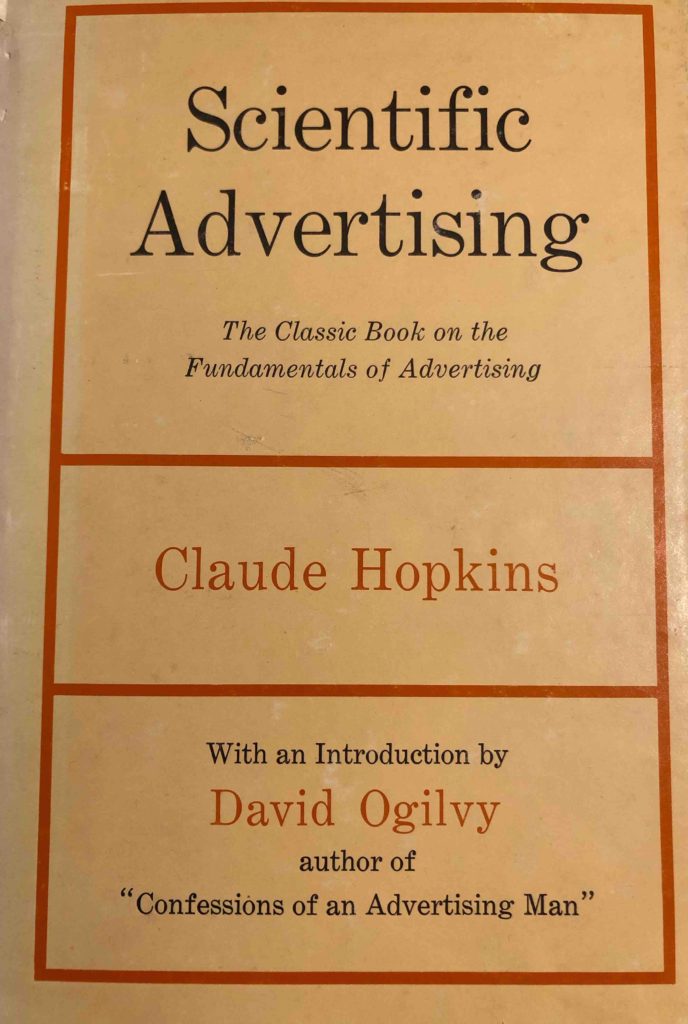
Claude Hopkins (1866-1932) wrote a book in 1923 called Scientific Advertising that was only 95 pages long but contains a large number of important insights about analytics that are just as available today as they were in 1923.
I have the 1966 edition by Crown Publishers that contains an introduction by David Ogilvy, who writes: “Nobody, at any level, should be allowed to have anything to do with advertising until he has read this book seven times.”
As Ogilvy points out, not everything in the book has stood the test of time, and some parts of the book were misguided when the book was published in 1923, but the majority of his insights are still worth following.
Hopkins was one of the earliest advocates of the Test-Measure-Refine (TMR) Loop in advertising. He was a strong advocate of coupons since coupons are not only an effective technique themselves but could be keyed, and the different keys could be used in the TMR Loop to measure outcomes of different variants of the ads. In 1923, the TMR mail-based loop required weeks, today we can do it in minutes to hours. It’s also important to note that the Test-Measure-Refine loop is also just as important in building analytic models as it is in advertising.
- [I spend] far more time on headlines than on writing. [I often spend] hours on a single headline [page 34].
- Almost any question can be answered cheaply, quickly and finally, by a test campaign. And that’s the way to answer them — not by arguments around a table [page 77].
- It is not uncommon for a change in headlines to multiply returns from five to ten times over [page 35].
- The best ads ask no one to buy. This is useless. Often they do not quote a price. … They offer wanted information. They cite advantages to users [page 23].
All quotes are from: Claude Hopkins, Scientific Advertising, Crown Publishers, Inc., 1966.
Hopkins wrote outstanding ad copy, including the slogan that Schlitz is the “beer that made Milwaukee famous.” His advertising and branding proficiency also created the brand equity for Palmolive soap and Pepsodent toothpaste.
In addition to stressing the fundamental importance of testing copy, Hopkins also pioneered the use of split testing, using coupons to provide samples and to track outcomes, loyalty programs, brand images, and product demonstrations in advertising. He was always testing and experimenting with new advertising ideas, which seems to be the reason why he titled his 1923 book Scientific Advertising.
Hopkins expertise and ability to execute was well appreciated. Indeed, he was hired in 1908 at the age of 41 to write ad copy for Lord & Thomas, at a salary of $185,000, the equivalent of over $5 million in 2019.
Hopkins influence and the influence of Lord & Thomas is still present even today. Lord & Thomas was founded in Chicago in 1873, and grew under the leadership of Albert Lasker to become in 1908 one of the largest general advertising firms in the US. When Lasker retired in 1943, he sold the firm to the executives who ran its three offices: Emerson Foote in New York, Fairfax Cone in Chicago, and Don Belding in Los Angeles and the firm became known as Foote, Cone and Belding. It’s still around today as FCB and still headquartered in Chicago.
The post is adapted from Chapter 4 of my book The Strategy and Practice of Analytics, copyright 2020.