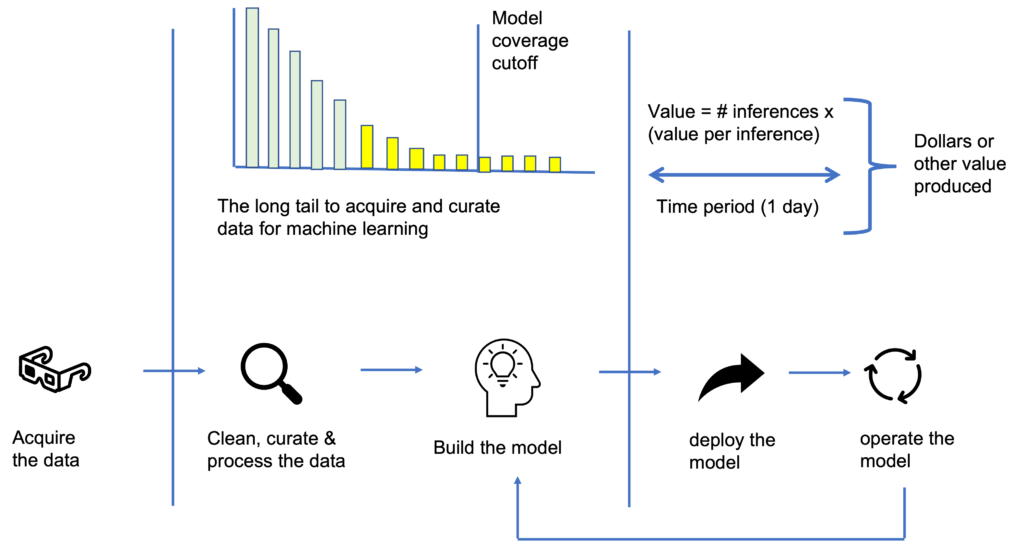
When developing an analytic strategy, it is important to have a good understanding of the economics of inferencing.
Our conceptional model is that we: 1) collect, purchase or otherwise acquire the data, 2) clean, curate and process the data so that it can be used as the inputs to models and to train models, 3) build the model; 4) deploy the model, and 5) operate the model, using the data as model inputs to to produce outputs of interest. See Figure 1.
To simplify the analysis, we will call the outputs of the model “inferences,” and assign a monetary value to each inference.
At the highest level, the economics of inferencing is driven by two equations: The first equation is the value provided by the inferences. This is the simplest equation and since inferences usually have a fixed value or a value determined by the subsequent actions (such as the value of the sale or value of the sale and subsequent sales over the next month). The value is simply the number of inferences x the value of each inference. This the right side of Figure 1.
The second equation is the cost of the inference. This is the sum of five components. See Table 1 and Figure 1. There is the cost to acquire the data, to clean and process the data, build the model, to deploy the model and to operate the model.
The challenge is that the cost to clean and process the data can have a long tail. In other words, to clean 50% of the data is often fairly easy, to clean 75% much hard, to clean 90% much, much harder, and, so, with the costs to clean the last few percent getting higher and higher.
There is an important difference between how for profit-companies offering a product or service and not-for-profit companies that are mission driven can manage the escalating cost associated with the long tail of data curation. For-profit companies can cut the tail at a sensible operating point, while not-for-profit or mission-driven organizations often have to process more of the tail in oder to satisfy their mission. Here are some example of why the long tail of data curation is often important for mission driven organizations:
- When cleaning up scientific data for machine learning a high model coverage threshold is important, since an important discovery may be in the long tail
- When identifying threats in national defense, a high model coverage threshold is important since missing just one threat can have devastating consequences
- When identifying fires and floods, covering the long tail is critical, since early detection of a fire or flood can result in significant savings of property and sometimes savings in lives.
costs of inferencing = cost to acquire the data large & variable + cost to clean & curate the data large & variable + cost to build the model fixed cost + cost to deploy the model fixed cost + cost to operate the model variable, but modest Table 1. Costs of inferencing
For more about the costs of acquiring and curating data, see Chapter 6 of Developing an AI Strategy: a Primer.