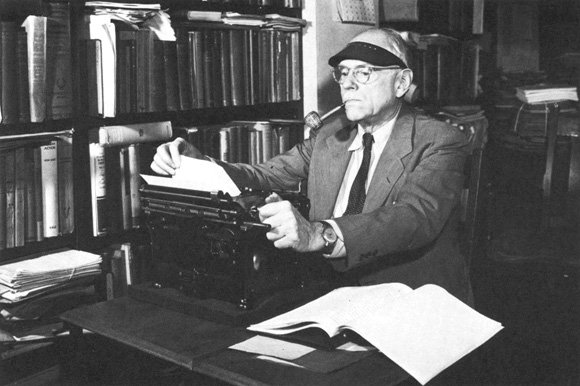
I have written several short profiles in this blog about individuals that have contributed to the practice of analytics or made intellectual contributions that can be applied to the practice of analytics, including:
- Claude Hopkins: An Early Advocate of Test Measure and Refine
- George Heilmeier: Twelve Rules for a Chief Analytics Officer, which covers Heilmeier’s Catechism and Heilmeier’s Twelve Rules for a new CIO and adapts them to the duties of a Chief Analytics Officer
- William H. Foege: Why Great Machine Learning Models are Never Enough: Three Lessons About Data Science from Dr. Foege’s Letter
- In Chapter 8 of my book Developing an AI Strategy: a Primer, I also provide brief profiles of Kenneth R. Andrews (1916-2005) and his four step process for strategic planning; H. Igor Ansoff (1918–2002) and what is now called the Ansoff Matrix; and, Bruce D. Henderson (1915-1992) and the experience curve.
In this post, I want to discuss some of Frank Knight’s insights about risk [1] and how they can be applied to the practice of analytics today.
Frank Knight and the Chicago School of Economics
Frank Knight (1885 – 1972) was a professor of economics at the University of Chicago from 1928 to 1955, where he was one of the founders of what became known as the Chicago School of Economics. His students included three Nobel prize winners in economics: Milton Friedman, George Stigler and James M. Buchanan.
His intellectual interests were broad and stretched from economics to social policy, political history and philosophy. He taught courses in the history of economic thought, the relationship between economics and social policy, and was one of the founding faculty members of the University’s of Chicago Committee on Social Thought in the early 1940s [2]. He was (cross)-appointed as a Professor of Social Science in 1942, a Professor of Philosophy in 1945, and was named the Morton D. Hull Distinguished Service Professor in 1946 [2].
Frank Knight’s Book – Risk, Uncertainty and Profits
In this post, I would like to discuss the important distinction between risk and uncertainty that Knight introduced in his 1921 book Risk, Uncertainty and Profit [1], which was based on his PhD dissertation. (The book is now in the public domain.) One of his key insights was that perfect competition would not eliminate profits, since even with perfect competition different firms would make different judgements due to the presence of uncertainty, which he distinguished from risk.
Modeling risk vs planning for uncertainty. At the most basic level, phrased as we would describe it today, Knight distinguished risk and uncertainty this way: you are modeling risk, when you know the different variables, alternatives and outcomes and can estimate their probabilities. You are planning for uncertainty, when you do not know or cannot measure the relevant variables, alternatives and outcomes and are developing plans and mechanisms to deal with this uncertainty.
It is interesting to note that when Knight’s book was published in 1921, mathematics and econometric modeling had not yet dominated the field and his entire book (at least from a quick scan) does not contain a single equation, although I counted six graphs illustrating concepts such as supply and demand.
Modeling Risk vs Planning for Uncertainty
When modeling risk, you can use standard machine learning models. When planning for uncertainty, you need to consider different scenarios and try to create appropriate plans for those you can imagine and even those that are challenging to image. As an example, planning for uncertainty includes thinking about what today we we would call black swan events [3].
Another way to look at this distinction, is that risk is about objective probabilities about events that can be measured, while uncertainty is about subjective probabilities about events that have to be postulated. Both are important in the practice of analytics.
A third way to look at this distinction was described in his book using markets. If there is a market so that you can insure against some unknown outcome, it is risk; if there is not a market, it is uncertainty [1].
Knight viewed entrepreneurs as those willing to put with uncertainty in certain circumstances and and to manage it in search of profits [1].
As more and more complicated risk instruments were developed over the years, fewer outcomes could be classified as uncertainty. On the other, as more and more complicated risk instruments were introduced, uncertainty increased, fragility increased, and the number of unknown unknowns increased. This created situations in which markets could collapse, such as the 2008 financial collapse, with mortgage backed securities introducing their set of uncertainties.
Risk, Uncertainty and the Practice of Analytics
In last month’s post, I discussed the importance of distinguish between developing analytic models, developing a system that employs analytics, and growing an analytics business. Recall that I use the term analytics to include machine learning, data science, statistical modeling, and AI. Let’s apply Knight’s distinction between risk and uncertainty to analytics models, systems, and businesses.
Quantifying risk when developing models. I have found it helpful at times to apply Knight’s insight about the distinction between risk and certainty to the practice of analytics. When developing machine learning or AI models, it’s important to quantify the errors and risks associated with a model by understanding the stochastic nature its inputs, hidden variables, and outputs and being able to describe their probability distributions. It’s equally important to be able to estimate the confidence level of the parameters in the model.
Managing uncertainty when developing ML or AI systems. On the other hand when developing systems that use machine learning (ML) or AI, it’s important to use engineering best practices to reduce the impact of uncertainty. For example, a best practice is “fuzz the system” that you are building by sending noise as inputs for days on end to make sure that the system performs gracefully no matter what inputs are provided, even non-numeric inputs, not printing characters, etc. Although fuzzing is standard in testing software for security vulnerabilities, it is also an excellent method to test AI-based systems to make sure they perform well in practice. Another standard best practice is to exponentially dampen temporally varying input variables in a system so that the system provides approximately good predictions even when one or two temporally varying inputs are in error.
Managing uncertainty in ML or AI businesses. Finally, uncertainty is also critical in building profitable machine learning or AI businesses. Good business strategies are robust in the sense they survive not just contingencies that are likely and predictable, but also those in which there is not enough data to quantify the risk and those that involve outcomes that have not yet been observed. Today, we might talk about how a business can survive disruptive changes in a market, but this can also be thought of a type of what is sometimes called Knightian uncertainty.
References
[1] Knight, Frank Hyneman. Risk, uncertainty and profit. Houghton Mifflin, 1921. The book is in the public domain and a copy can be found here.
[2] University of Chicago Library, Guide to the Frank Hyneman Knight Papers 1908-1979, University of Chicago Frank Hyneman Knight Special Collection.
[3] Taleb, Nassim Nicholas. The black swan: The impact of the highly improbable. Random house, 2007.