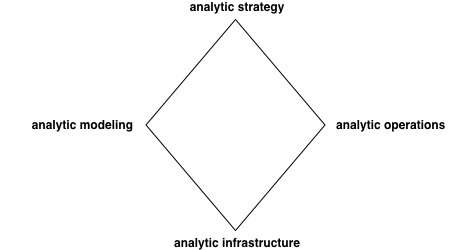
One of the most useful frameworks that I have found looks at a data science, machine learning or analytics project from four different perspectives:
Analytic modeling. The analytic model team consists of statisticians and data scientists that develop, test and validate the statistical or machine learning model.
Analytic infrastructure. The analytic infrastructure team consists of data engineers and those knowledgeable about databases, data science frameworks, enterprise IT and related areas and are responsible for the analytic and IT infrastructure required for managing the data required to build the models and managing the systems required to deploy the model into products, services and internal operational processes.
Analytic operations. The analytic operations team deploys the model into a product, service or internal operational process. Importantly, it is also responsible for integrating various actions associated with the outputs of the model and improving the actions so that they bring the required value. For example, a model might output a score from 1 to 1000, but depending upon the value of the score, no action may be taken, a standard offer may used, or a special offer may be used.
Analytic strategy. The analytic strategy team selects appropriate analytic opportunities and works with the product team to develop an appropriate business model for the product or service developed.
I’ll be talking at Predictive Analytic World about analytic operations:
Title: What are Analytic Operations and What Are Some Frameworks to Improve Them?
Abstract: There is a lot of information and best practices available so data scientists can build analytic models, but much less about how analytic models can best be integrated into a company’s products, services or operations, which we call analytic operations. We describe three frameworks so that a company or organization can improve its analytic operations and explain the frameworks using case studies.
Event: Predictive Analytics World, Las Vegas, June 19, 2019.